A new report published by the UC Berkeley Center for Long-Term Cybersecurity (CLTC) aims to help organizations develop and deploy more trustworthy artificial intelligence (AI) technologies.
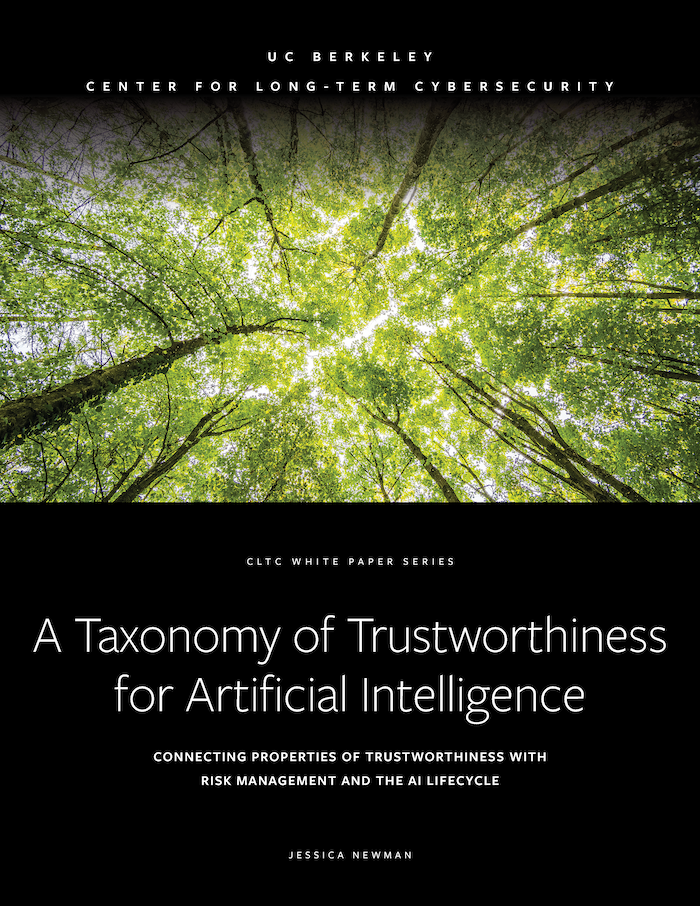
A Taxonomy of Trustworthiness for Artificial Intelligence: Connecting Properties of Trustworthiness with Risk Management and the AI Lifecycle, by Jessica Newman, Director of CLTC’s AI Security Initiative (AISI) and Co-Director of the UC Berkeley AI Policy Hub, is a complement to the newly released AI Risk Management Framework, a resource developed by the U.S. National Institute of Standards and Technology (NIST) to improve transparency and accountability for the rapid development and implementation of AI throughout society.
“This paper aims to provide a resource that is useful for AI organizations and teams developing AI technologies, systems, and applications,” Newman wrote. “It is designed to specifically assist users of the NIST AI RMF, however it could also be helpful for people using any kind of AI risk or impact assessment, or for people developing model cards, system cards, or other types of AI documentation.”
The NIST AI RMF defines seven “characteristics of trustworthy AI,” which include: valid and reliable, safe, secure and resilient, accountable and transparent, explainable and interpretable, privacy-enhanced, and fair with harmful biases managed. Using these characteristics as a starting point, the CLTC report names 150 properties of trustworthiness, which are mapped to particular parts of the AI lifecycle where they are likely to be especially critical. Each property is also mapped to specific parts of the AI RMF core, guiding readers to the sections of the NIST framework that offer the most relevant resources.
A key purpose of the report is to help developers of AI systems consider a broad range of questions to ensure they are deploying their technologies responsibly, such as: how will we verify that the system is behaving as expected? How will we ensure that a human is in control or meaningfully in the loop of the operational decision-making process of the AI system? How will we test the ability of the AI system to try to “game” a proxy of a true objective function, or to learn novel methods to achieve its objective function? And how will we ensure the AI system only presents outputs that are accurate and not intentionally deceptive?
“Each property of trustworthiness offers a distinct lens through which to assess the trustworthiness of an AI system and points to a set of questions and decisions to be made,” Newman wrote. “Many stakeholders have a role to play in developing and ensuring trustworthy AI. The process should include a broad range of roles from within an organization as well as outside experts, including members of impacted communities and independent verification and auditing bodies.”
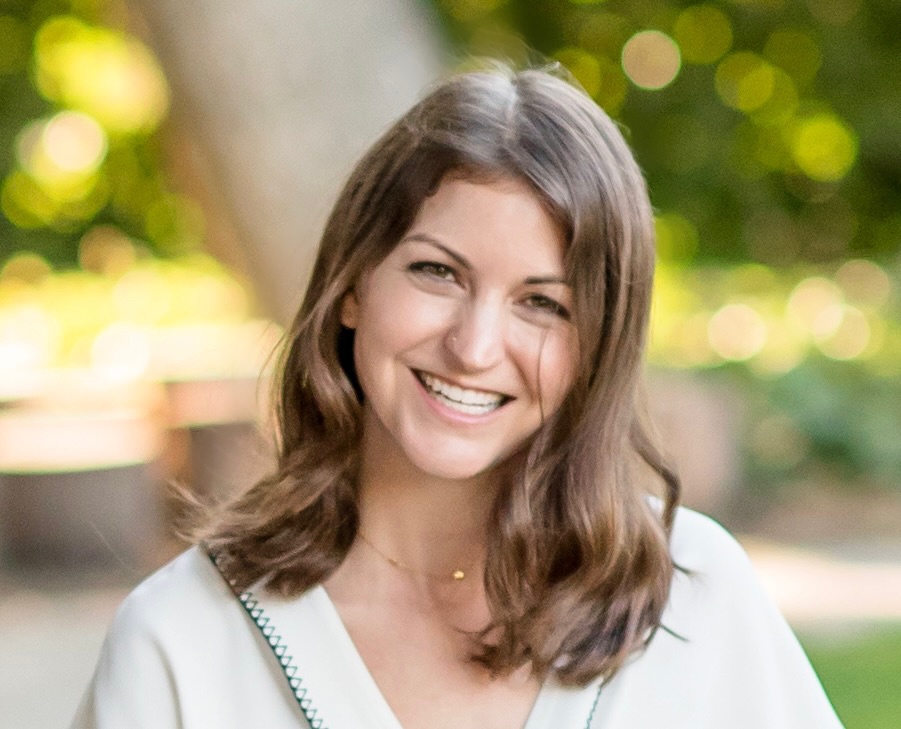
Unlike many resources focused on responsible AI, the new taxonomy considers AI-based systems that may operate without direct human interaction. “The taxonomy was developed to be useful for understanding a full spectrum of AI systems, including those that have limited engagement with people, which have typically been underemphasized in considerations of AI trustworthiness,” Newman wrote. “The paper includes further discussion of the spectrum of human-AI engagement and how this relates to trustworthiness.”
Issued as part of the CLTC White Paper Series, the report is the result of a year-long collaboration with AI researchers and multistakeholder experts. Newman developed the taxonomy based on an array of papers, policy documents, and extensive interviews and feedback, as well as an expert workshop that CLTC convened in July 2022.
The paper can help teams and organizations assess how they are incorporating properties of trustworthiness into their AI risk management process at different phases of the AI lifecycle. In addition to the NIST AI-RMF, the paper also connects the properties to select international AI standards, such as those issued by the Organisation for Economic Co-operation and Development (OECD), the European Commission, and the European Telecommunications Standards Institute (ETSI).
“The taxonomy may serve as a resource and tool for organizations developing AI, as well as for standards-setting bodies, policymakers, independent auditors, and civil society organizations working to evaluate and promote trustworthy AI,” Newman explained. “We hope it serves as a useful resource alongside the NIST AI RMF, helping users to further explore the relationships between NIST’s core framework, characteristics of trustworthiness, and depiction of the AI lifecycle.”