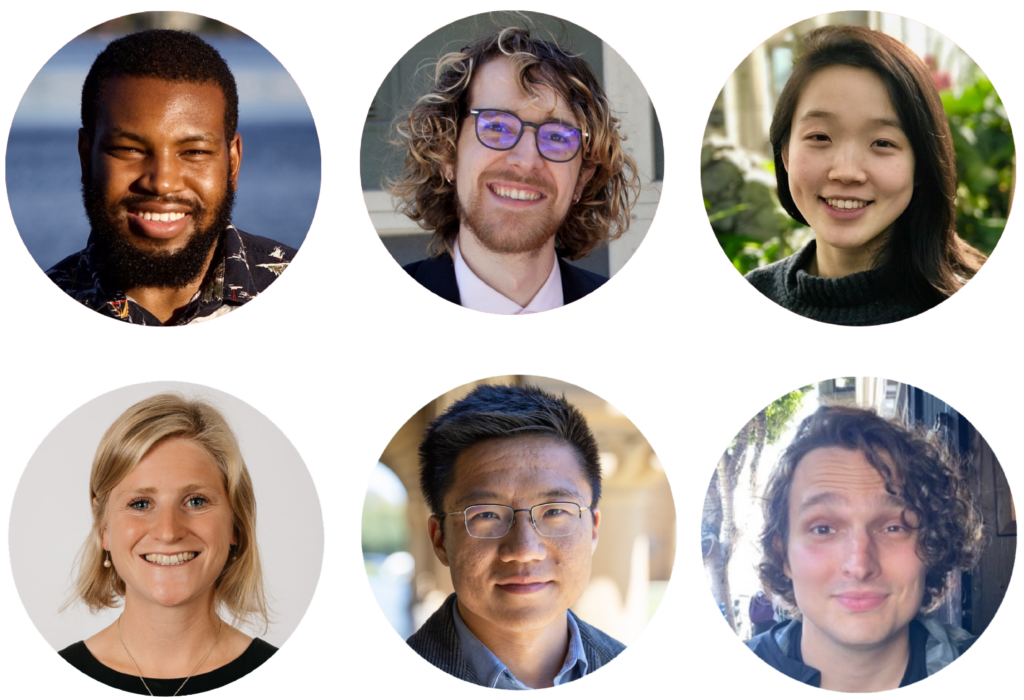
The AI Policy Hub is an interdisciplinary initiative at UC Berkeley dedicated to training graduate student researchers to develop governance and policy frameworks to guide artificial intelligence, today and into the future.
On April 20, 2023, the AI Policy Hub held its inaugural AI Policy Research Symposium, a showcase of the research carried out by the initial cohort of AI Policy Hub Fellows, a group of six graduate students whose work addresses topics such as improving the explainability of AI systems, understanding the consequences of AI-enabled surveillance, and minimizing the harms of AI-based tools that are increasingly used in criminal prosecutions.
Below are summaries of each researcher’s work, along with a link to the video of their presentation. Follow the hyperlinks to jump to any of the profiles.
To view the entire AI Policy Hub Research Exchange, including keynotes by Stuart Russell and Pam Samuelson, please visit this recap: https://cltc.berkeley.edu/2023/05/09/uc-berkeley-ai-policy-research-symposium/
- Alexander Asemota: Using counterfactuals to improve explainability in machine learning
- Zoe Kahn: Understanding how communities may be affected by AI and machine learning
- Micah Carroll: Researching the impacts of recommenders based upon reinforcement learning (RL)
- Zhouyan Liu: Studying AI-enabled digital surveillance in China
- Angela Jin: Investigating the use of AI-based evidence in the US criminal legal system
- Cedric Whitney: Understanding algorithmic disgorgement and the “right to be forgotten” in AI systems
Alexander Asemota
Using counterfactuals to improve explainability in machine learning
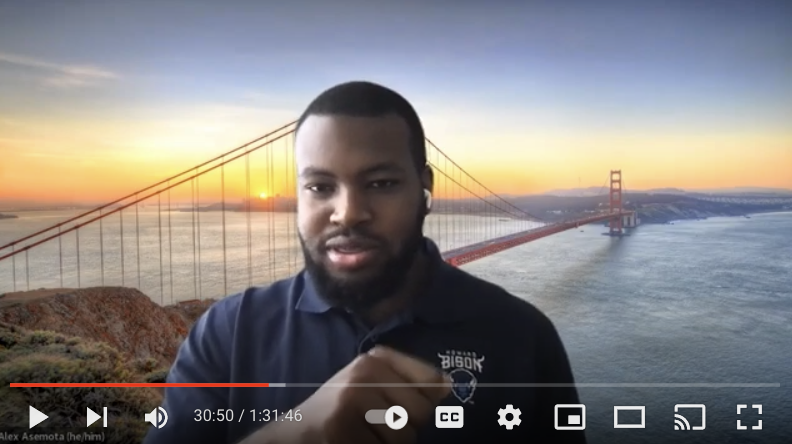
Alexander Asemota, a third-year PhD student in the UC Berkeley Department of Statistics, discussed his research on explainability in machine learning. He explained that he is investigating how “counterfactuals” — statements about something that did not happen, rather than what did — could be an effective approach for explaining the outputs of artificial intelligence.
AI is “increasingly used to make decisions in high-stakes contexts, for example, approving or denying someone for a loan,” Asemota explained, but “systems use complicated models that no one may understand. Even if the developer understands a model, how would they explain those decisions to someone who has no background in AI? Counterfactual explanations are a promising solution to this problem.”
“A counterfactual can answer the question, what if I had done x?” he said. “For example, if I apply for a loan, and I was denied, I can ask, if my income had been $1,000 higher, would I have been accepted for the loan? This approach can tell someone what they need to do to get the solution they want, and provides actionable feedback.”
His research has diverse policy implications, Asemota said. “Organizations that use counterfactuals for explanations should compare the recommendations to observed changes,” he said. “This improves the feasibility of recommendations, and it assists in differentiating between multiple different recommendations. So you can see which recommendations are more likely than others, based on observed changes in the past…. And though counterfactuals have significant potential, regulators really should scrutinize their use to make sure that they aren’t violating regulations. With that in mind, I’ve been working on a white paper directly to the Consumer Financial Protection Bureau, suggesting AI and explanation.”
Zoe Kahn
Understanding how communities may be affected by AI and machine learning
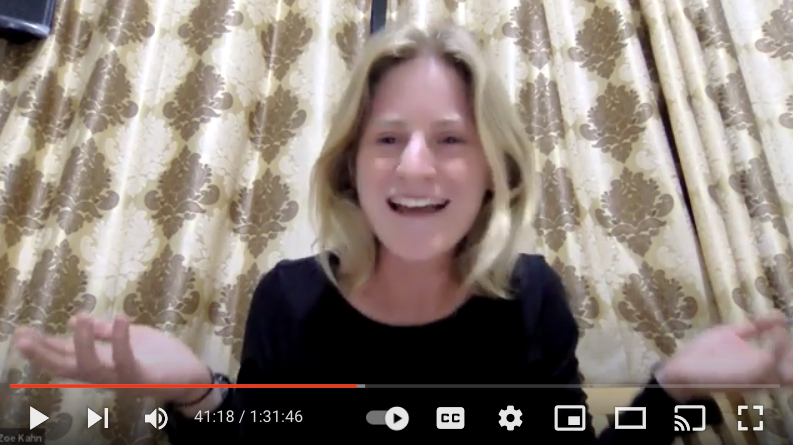
AI Policy Hub Fellow Zoe Kahn presented her research using qualitative methods to understand the perspectives and experiences of communities that may be negatively impacted by AI and machine learning technologies. Kahn is working on a project that uses data-intensive methods to allocate humanitarian aid to individuals experiencing extreme poverty in Togo. Her research is related to a digital cash aid program in Togo that provides money digitally, with individuals’ eligibility determined through automated methods.
“The criteria that was used to determine who would receive money from this program (and who would not) was based on a machine-learning model that uses mobile phone metadata,” she said. “What we were really interested in understanding were the perspectives and experiences of people living in rural Togo who are really impacted by by this particular program. These are often people who have no technical training, little to no formal education, and varying levels of literacy.”
Kahn sought to provide subjects in her study with an “effective mental model about the data that’s recorded by mobile phone operators, as well as an intuition for how that information could be used to identify people in their community who are wealthier, and people in their community who are poor.” She and her team developed a visual aid to convey this understanding, and found it was “really useful as a way of providing people with an explanation.”
“Participants took up elements of the visual aid during the interviews both as a way of answering our questions, but also as a way of articulating their own ideas in new and interesting ways,” she said. “Not only do these methods enable policymakers to engage in what I’d call ‘good governance,’ but in my experience doing this work in Togo, it really is a way to treat people with dignity. My hope is that the methods we have developed in Togo can be used as a way to really meaningfully engage people who may not have technical training or familiarity with digital technology.”
Micah Carroll
Researching the impacts of recommenders based upon reinforcement learning (RL)
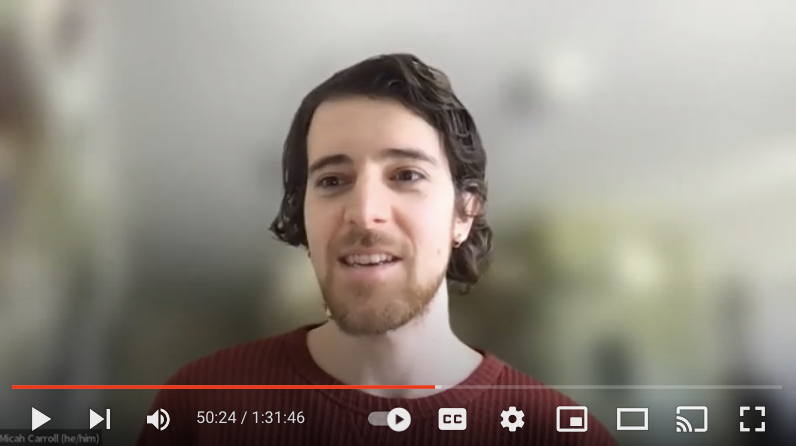
Micah Carroll, a third-year Artificial Intelligence PhD student, presented his research on recommender algorithms, software used in a variety of everyday settings, for example, to recommend content on Netflix and Spotify or display products on Amazon based on a user’s past behavior. “One thing that’s understudied in this area is that user preferences are subject to change, and recommender algorithms themselves may have incentives to change people’s preferences — and their moods, beliefs, behaviors, and so on,” Carroll said.
Carroll studies the impacts of recommenders that are based upon reinforcement learning (RL), which are designed to “modify the state of the environment in order to get high rewards.” The use of RL creates “clear incentives for the recommendation system to try to manipulate the user into engaging more with the content they’re shown, given that the recommender system is being rewarded for keeping users engaged on the platform for longer,” he explained. “The question remains, can real recommender systems actually manipulate users? And I think there’s definitely a variety of evidence that shows that this seems in fact quite plausible.”
Companies should increase their transparency for AI systems that interface with humans, and describe in more detail what types of algorithms they’re using and how they’re using them, Carroll said. He also proposes the use of “auditing pathways” to enable routine auditing by third-party auditors, as well as “manipulation benchmarking standards” that can help measure the impacts. “One of the most important insights of this line of work is that manipulation can happen even without designer intent,” he said. “We should be worried about misuse and intentional manipulation that individuals might try to enact, but even companies that are for the most part trying their best might engage accidentally in manipulation.”
Zhouyan Liu
Studying AI-enabled digital surveillance in China
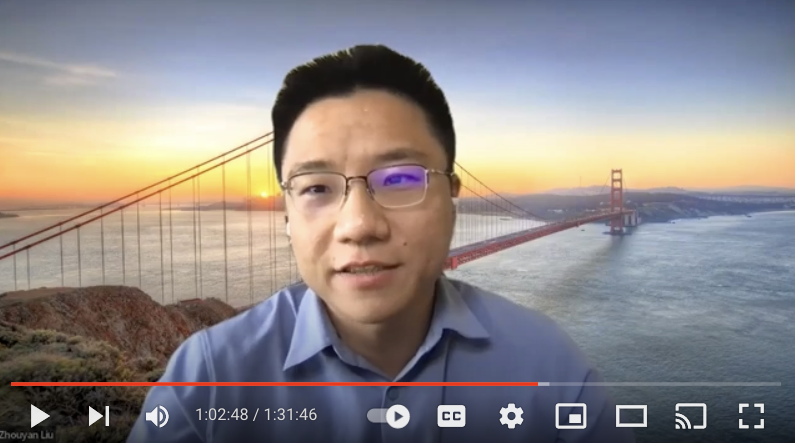
The next presentation was by Zhouyan Liu, a former investigative journalist who is now a student in UC Berkeley’s Master of Public Policy degree program, conducting empirical studies on China’s technology policy, digital surveillance, and privacy.
Liu said that China’s “surveillance actions raise our concerns about basic human rights and privacy issues,” but he emphasized that “surveillance itself is in fact one of the oldest political actions, not limited to China or the present day…. My question is, when the oldest political practice meets the latest technology, what has changed? What exactly has a technology changed about surveillance, and what impact has it had?”
China’s government relies on surveillance, Liu explained, because “prevention is better than the cure,” as technology empowers authoritarian rulers to detect problems and potential threats before people resist, and it provides the “manpower needed to collect large amounts of data” and the “ability to efficiently and comprehensively aggregate data about the same individuals from different fields and departments and perform automatic analysis.” His study sought to determine whether China’s government uses surveillance to limit unrest, and whether it improves regime security, by reducing the number of protests or reducing ordinary criminal offenses.
Using data drawn in part from the Chinese government’s pilot of the Sharp Eyes surveillance program, Liu concluded that AI-based surveillance did increase the government’s ability to intervene in civil disputes, but did not result in a significant change in the number of political opponents arrested. And while civil protests decreased, crime rates remained unchanged.
“The implication of the study is first and foremost significant for individuals living in authoritarian countries,” Liu said. “Even if you do not express any dissatisfaction or protest against the current regime, you’re still being monitored automatically by the system. On the other hand, such a system actually has a huge impact on the structure of authoritarian governments themselves. Some grassroots officials explicitly told me that they do not like this AI system, because AI technology takes away the power that originally belonged to them, and decisions and data are made at higher levels. This will be a shock to the entire government structure, and what political consequences they will bring is still unknown.”
Angela Jin
Investigating the use of AI-based evidence in the US criminal legal system
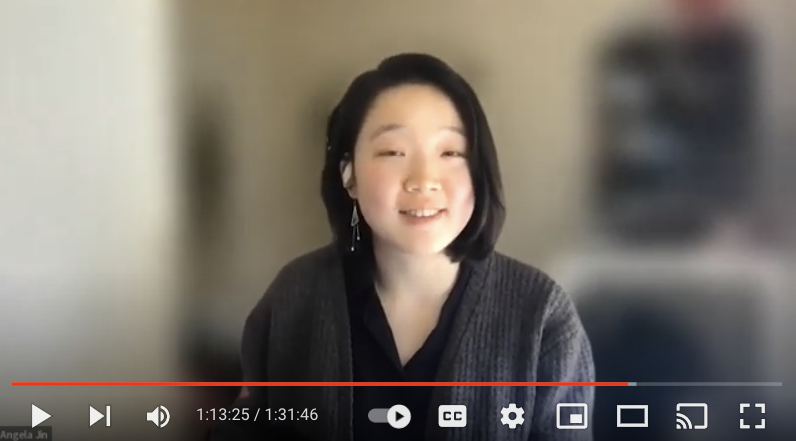
Angela Jin, a second-year PhD student at UC Berkeley, presented a study on the use of evidentiary AI in the US criminal legal system, focused on the use of probabilistic genotyping software (PGS), which is increasingly used in court cases to prosecute defendants, despite not having been properly validated for accuracy. PGS is used to link a genetic sample to a person of interest in an investigation.
“These cases have led to many calls for an independent assessment of the validity of these software tools, but publicly available information about existing validation studies continues to lack details needed to perform this assessment,” Jin said. “Despite these concerns, PGS tools have been used in a total of over 220,000 court cases worldwide. And evidentiary software systems are not just being applied to DNA evidence. Tools have been developed in use for many other applications, such as fingerprint identification, gunshot detection, and tool mark analysis.”
Jin’s study focused on internal validation studies, a series of tests that a lab conducts on the PGS prior to using it in casework. “These studies are commonly referred to in courtroom discussions, along with the standards that guide them, as indicators of PGS reliability and validity,” she said. “But these standards provide ambiguous guidance that leaves key details up to interpretation by individual forensic labs…. Second, these standards do not account for the technical complexity of these systems.”
She explained that she and her collaborators are creating a testing framework for probabilistic genotyping software. “In this work, our goal is to eventually influence testing requirements while creating more rigorous testing standards will help us move toward more reliable use of PGS in the US criminal legal system,” she said. “My work also seeks to incorporate defense attorney perspectives into the creation of such testing requirements and policies, especially as policymakers increasingly seek to incorporate perspectives from diverse stakeholders….Together, these projects seek to influence policy as a way to move us towards responsible use of PGS.
Cedric Whitney
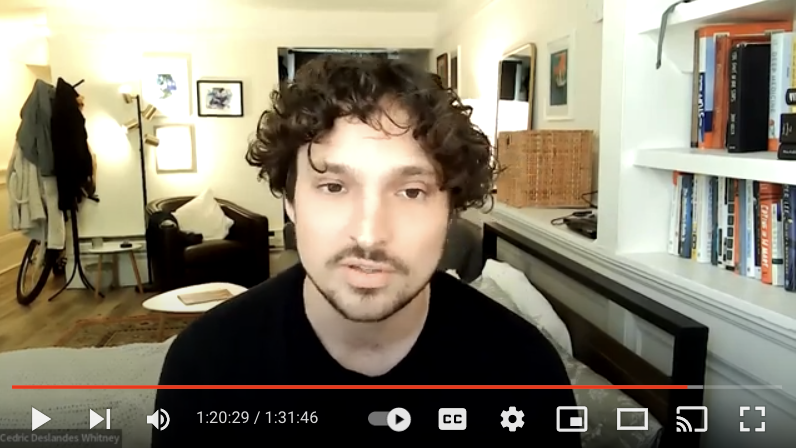
The final AI Policy Hub Fellow to present was Cedric Whitney, a third-year PhD student at Berkeley’s School of Information whose research focuses on using mixed methods to tackle questions of AI governance. He presented research building on prior work he carried out at the Federal Trade Commission (FTC) focused on algorithmic disgorgement and the “right to be forgotten” in AI systems.
Whitney explained that algorithmic disgorgement is “a remedy requiring a party that profits from illegal or wrongful acts to give up any profit that they made as a result of that illegal or wrongful conduct. The purpose of this is to prevent unjust enrichment and to make illegal conduct unprofitable. The FTC recently began using this remedy as a novel tool and settlement with companies that have developed AI products on top of illegally collected data.”
A related challenge, Whitney explained, is that “when a company builds a product on top of illegally collected data, just enforcing that they delete that data doesn’t actually remove the value they derive from it. A model trained on data doesn’t suddenly self-destruct itself post data deletion. By requiring that a company also delete the effective work product, algorithmic disgorgement takes aim at the true benefit of illegal data collection in these settings. My research has been looking at the landscape for use of this tool.”
Through his research, he hopes to “create a resource for policymakers and technologists to better understand what algorithmic disgorgement can and cannot do, both to assist in functional implementation, and prevent it from being portrayed as a panacea to all algorithmic harm…. It should be a priority to increase regulatory funding to meet the hiring needs necessary for utilizing this and other governance tools,” as “there is a high burden on technical capacity to even begin utilizing them at an effective manner.”