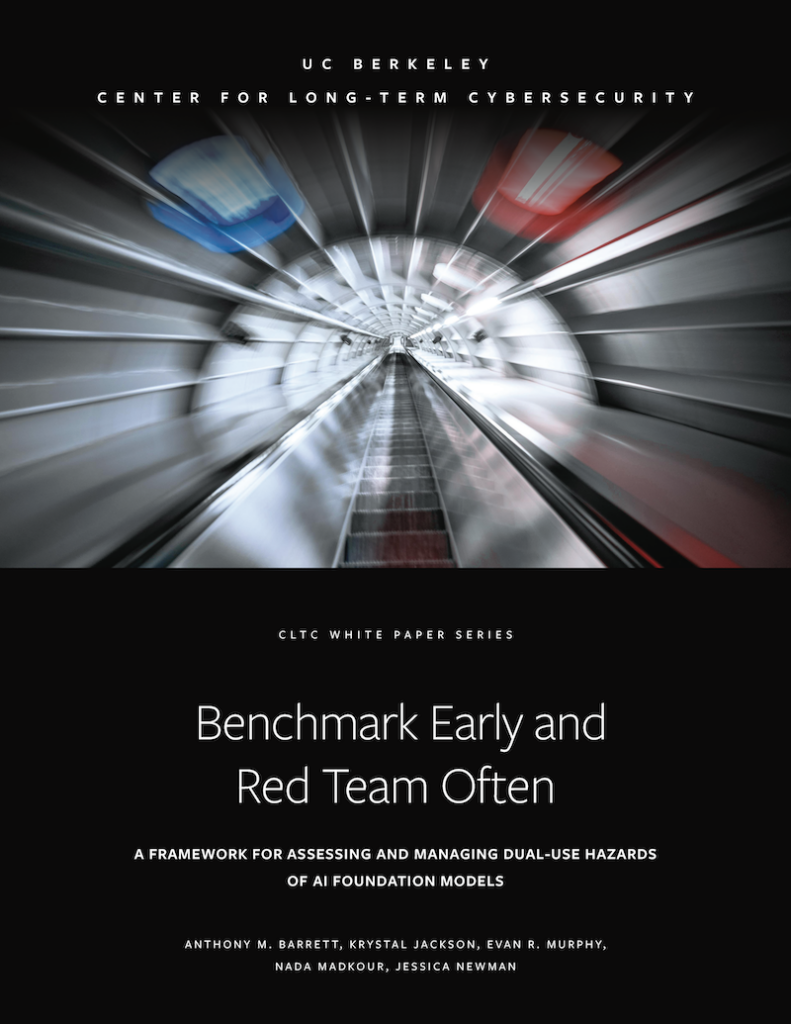
How can developers of artificial intelligence (AI) ensure that their models cannot be used by a terrorist, state-affiliated threat actor, or other adversary to develop chemical, biological, radiological, or nuclear (CBRN) weapons, or to carry out a major cyberattack?
A new report by a team of researchers from the Center for Long-Term Cybersecurity and AI Security Initiative addresses this critically important question. The paper, Benchmark Early and Red Team Often: A Framework for Assessing and Managing Dual-Use Hazards of AI Foundation Models — authored by Anthony M. Barrett, Krystal Jackson, Evan R. Murphy, Nada Madkour, and Jessica Newman — assesses two methods for evaluating the “dual-use” hazards of AI foundation models, which include large language models (LLMs) such as GPT-4, Gemini, Claude 3, Llama 3, and other general purpose AI systems.
The first approach relies on the use of open benchmarks, sets of questions that can be used to query an AI model to assess whether it will share details necessary to develop a CBRN or cyber attack. Such benchmarks are low in cost, the authors explain, but have limited accuracy, as the questions and answers used in publicly available benchmarks should not include security-sensitive details. Thus, using benchmarks alone may make it difficult to discern models with “general scientific capabilities” as opposed to models “with substantial dual-use capabilities for CBRN or cyber attacks.”
The second approach relies on using closed red team evaluations, in which teams of experts on CBRN and cyber assess a model’s potential to disclose potentially harmful information. Red teams are higher in cost than benchmarks, the authors write, as they “require specialized expertise and take days or weeks to perform,” but they can achieve higher levels of accuracy, as the experts on the red team can incorporate sensitive details in their assessments.
“We propose a research and risk-management approach using a combination of methods including both open benchmarks and closed red team evaluations, in a way that leverages advantages of both methods,” the authors write. “We recommend that one or more groups of researchers with sufficient resources and access to a range of near-frontier and frontier foundation models: 1) run a set of foundation models through dual-use capability evaluation benchmarks and red team evaluations, then 2) analyze the resulting sets of models’ scores on benchmark and red team evaluations to see how correlated those are.”
The authors argue that open benchmarks should be used frequently during foundation model development as a quick, low-cost measure of a model’s dual-use potential. Then, if a particular model gets a high score on the dual-use potential benchmark, more in-depth red team assessments of that model’s dual-use capability should be performed. They call this approach the “Benchmark and Red team AI Capability Evaluation (BRACE) Framework,” summarized as “Benchmark Early and Red Team Often,” or more accurately, “Benchmark Early and Often, and Red Team Often Enough.”
The report includes recommendations for three distinct groups: researchers who create benchmarks and red teaming evaluations of CBRN and cyber capabilities of foundation models, developers of foundation models, and evaluators of dual-use foundation models. The paper additionally discusses limitations and mitigations for the BRACE approach, for example, if model developers try to game benchmarks by including a version of benchmark test data in a model’s training data.
This paper aims to advance the conversation by providing greater clarity around some existing and near-term risks of dual-use foundation models that can be used to cause significant harm to people and communities,” the authors write. “The research we propose could inform subsequent use of benchmarks and red teaming by foundation model developers and evaluators, with an approach to effectively and efficiently identify foundation models with substantial dual-use capabilities for risk management purposes.”