On April 26, 2024, the UC Berkeley AI Policy Hub presented the second-annual AI Policy Research Symposium, a showcase of cutting-edge AI research from across the UC Berkeley community. The 90-minute event featured keynote presentations from faculty members Niloufar Salehi and Ken Goldberg, as well as presentations from the Spring 2023-Fall 2024 AI Policy Hub fellows.
The AI Policy Hub is an interdisciplinary initiative that trains researchers to develop effective governance and policy frameworks to guide artificial intelligence, with a goal to help policymakers and other AI decision-makers act with foresight. The Hub is jointly operated by the AI Security Initiative (AISI), a program within the Center for Long-Term Cybersecurity (CLTC), and the CITRIS Policy Lab, part of the Center for Information Technology Research in the Interest of Society and the Banatao Institute (CITRIS).
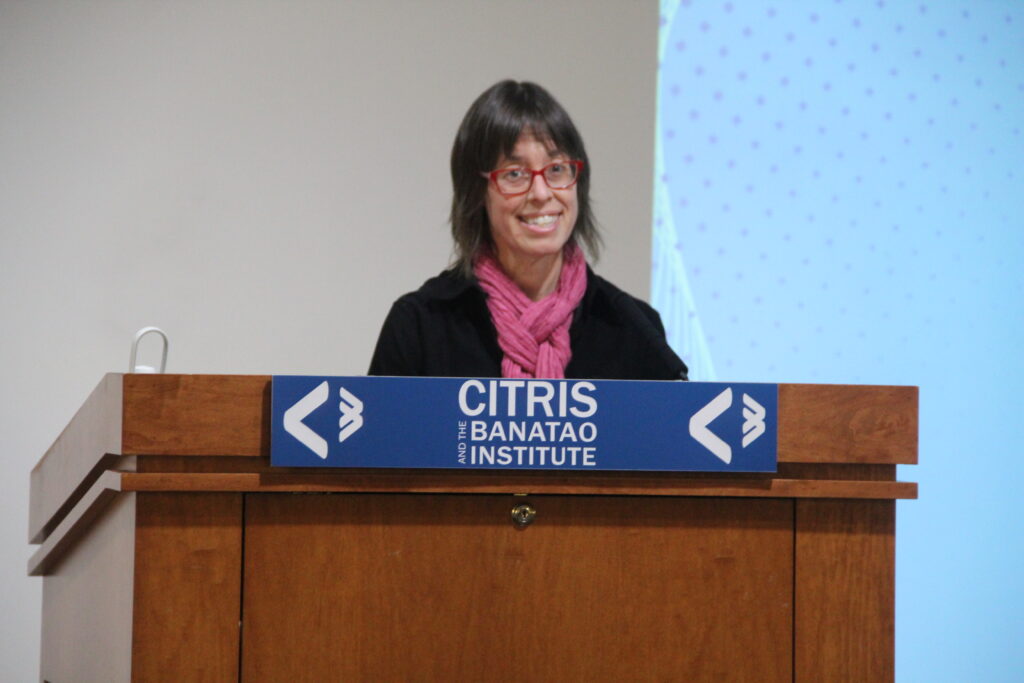
“CLTC works to look at the future of cybersecurity and to expand who participates in cybersecurity, and I can’t think of any program more emblematic of that mission than the AI Policy Hub,” said Ann Cleaveland, Executive Director of CLTC, in her introductory remarks. “The AI Policy Hub has grown into this incredible community that’s contributing cutting-edge research and developing the next generation of bridge-builders between AI technology and AI policy, folks who will be leaders in the field of AI governance and safe and beneficial AI for years to come.”
The symposium was moderated by Jessica Newman, Director of the AISI and Co-Director (with Brandie Nonnecke) of the AI Policy Hub. “Our vision for the AI Policy Hub was to create a space on campus to take people out of their academic silos and provide meaningful support for research into some of the thorniest AI policy challenges,” Newman explained.
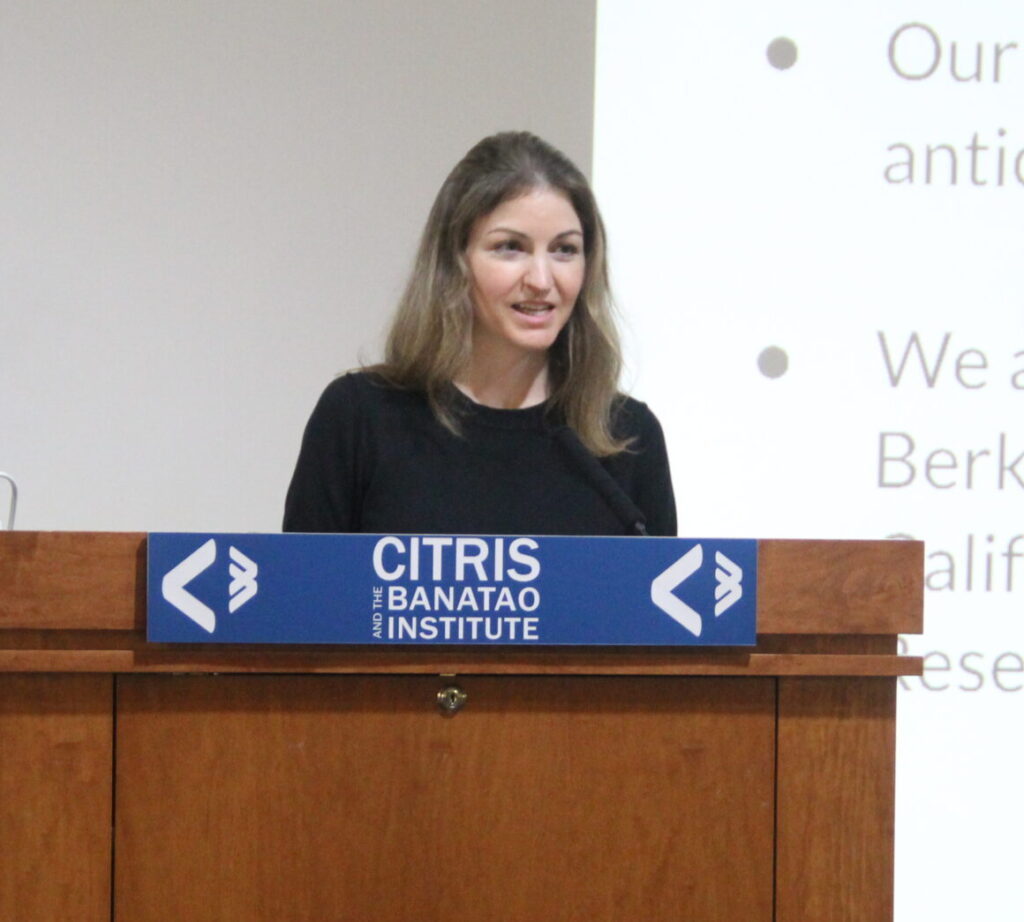
“We select annual cohorts of UC Berkeley graduate students following a competitive application process and provide these students with research fellowships for a full academic year. The cohort then has the opportunity to not just further their own research, but to learn from peers with different expertise and to benefit from hands-on training, workshops, speaker series, and other events. The Fellows learn the skills to translate their academic work for policymakers and other decision-makers, and are well-positioned to move into impactful careers in the AI policy field.”
Keynote: Niloufar Salehi
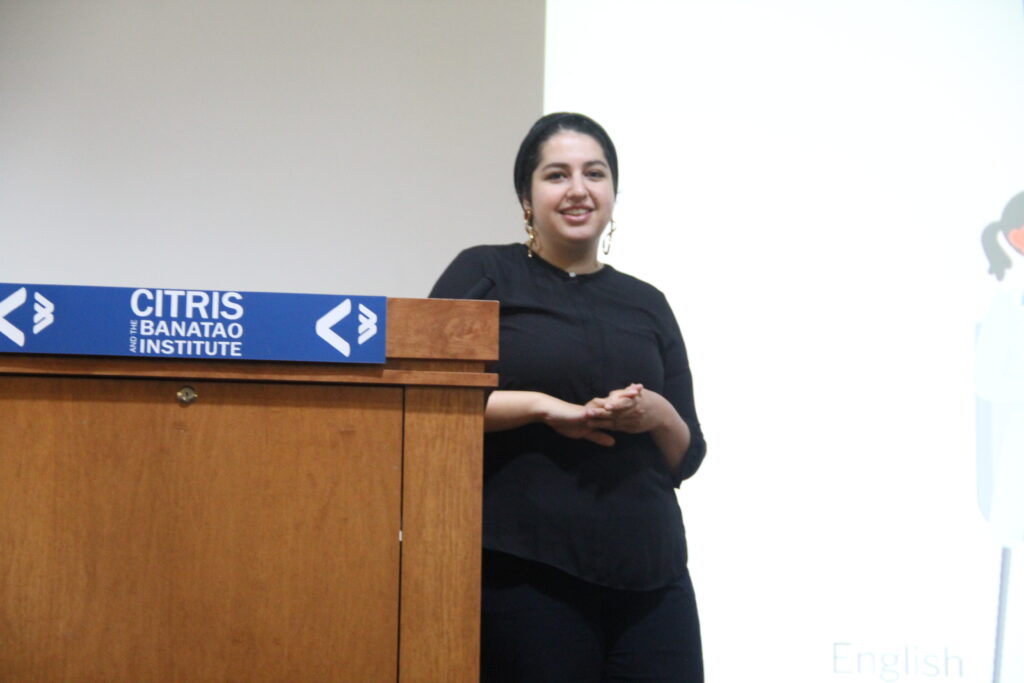
The first keynote was presented by Niloufar Salehi, Assistant Professor in the UC Berkeley School of Information. Salehi’s research focuses on social computing, human-centered AI, and more broadly, human-computer interaction, spanning fields such as education, health care, and restorative justice.
“Over the past two years, being part of the AI Policy Hub has been really a highlight of being at Berkeley,” Salehi said. “We have so many open questions when it comes to AI and society and policy, and I don’t think that it’s possible to even start to approach them if we don’t take this interdisciplinary approach that the AI Policy Hub has been cultivating.”
Salehi’s talk centered on a central challenge for shaping policy in the future: the unreliability and inconsistency of AI. She provided an example of doctors who use Google Translate to communicate with patients who don’t speak English, noting that they cannot always verify the accuracy of the translations, leading to potentially fatal errors. “What is happening on the ground is that doctors are making these cost-benefit analyses,” Salehi said. “What is the risk of this being an incorrect translation, versus what is the potential risk of this person not getting this information in time?”
She noted that the Google Translate interface is part of the problem. “It’s really hard to identify errors,” she explained, noting that the interface looks the same regardless of whether the accuracy of the output is high or low. “There are no actionable strategies to recover from errors. What has that got to do with policy? A lot of what we do end up doing when we’re making policy for systems that don’t always work is that we provide guidelines for professionals and experts who are using those tools. And if we don’t have good ways to create guidelines, we don’t have good ways to create good policy.”
Salehi stressed that AI systems often present “uncertainty around capabilities, output, complexity, and unpredictable behavior in different conditions.” She explained that her research focuses on developing methods of evaluating model outputs, helping people craft good inputs to models, and using “restorative justice” to address the harmful impacts of AI. “We need to be really thinking about design for reliability,” she said. “We need some hybrid systems that have some form of a machine learning model, together with some external sources of verifiable information, so that we can have that groundedness and reliability. And we need to do a ton more work on evaluation.”
AI Policy Fellows: AI Evaluation and Auditing
The next section of the symposium featured brief “lightning talks” by the Fall 2023-Spring 2024 AI Policy Fellows. The talks were broken down into three categories: AI evaluation and auditing, responsible use, and accountability for harms. “Being able to investigate the AI models that are in use is absolutely critical so that we have greater transparency into what models are actually in use, how they might fail under different circumstances, and how those failures might impact different communities,” Newman explained.
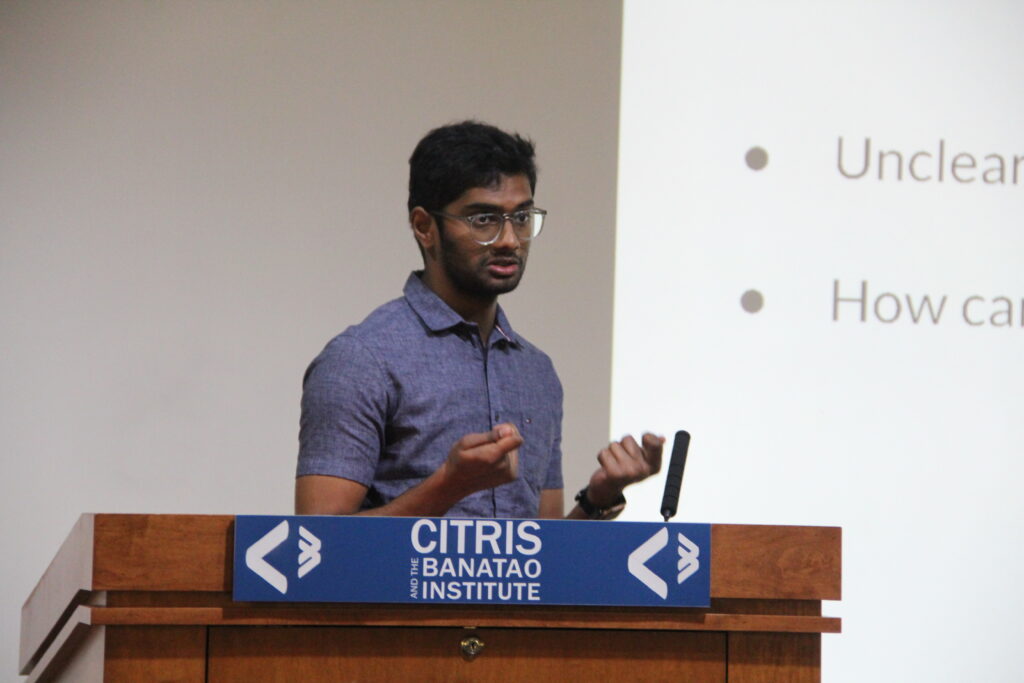
In his talk, Guru Vamsi Policharla, a PhD student in the UC Berkeley Department of Electrical Engineering and Computer Sciences (EECS), presented his research, “Zero Knowledge Proofs for Machine Learning.” This work focuses on the potential of cryptographic proofs of training to produce publicly verifiable proofs that an AI system is robust, fair, valid, and reliable, without compromising the privacy of the underlying dataset or the machine learning model. This tool can be used to support accountability from companies deploying AI, especially those that limit public access on their training procedures and datasets.
“Everybody agrees more or less that we really need regulation around AI systems and nobody should be deploying them haphazardly, but it’s very unclear how you can be sure that people are actually regulation-compliant,” Policharla said. “How can you actually be sure that the model that was certified is being used?”
Policharla explained that using cryptographic “zero-knowledge” proofs allow for robust evaluation of a model without revealing private data. “What this proof of training is going to say is, hey, I took this data set and I actually trained on this data set to arrive at this model, but you’re not going to reveal the model or the data set. That sounds like magic, but that’s what cryptography lets you do.”
He said that, while the solution is limited to small to medium-sized models, “it really is possible to enforce regulation compliance without the need for a middleman. And once you set up the program, it just happens in the background, even when the model gets updated, and fixes itself. You don’t need to bring auditors back and forth, and it’s a very smooth system once you get it up and running.”
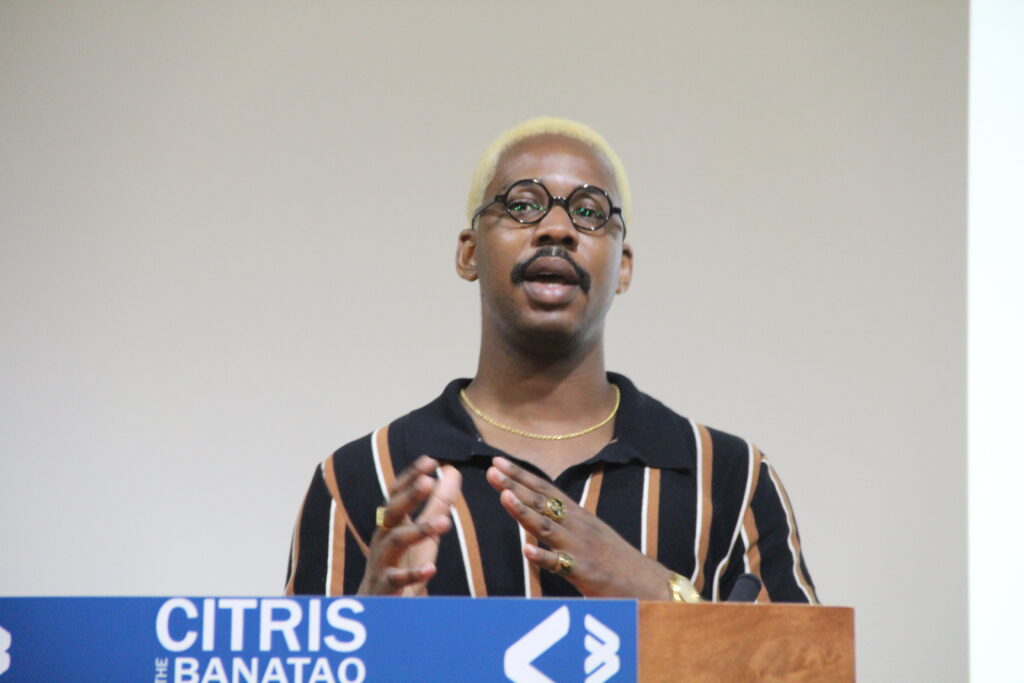
The next talk was by Christian Ikeokwu, a PhD student in the EECS department who researches the risk that users may intentionally or unintentionally bypass the safety mechanisms of generative AI models, leading to unintended and potentially harmful outcomes. He is developing algorithms to teach AI general safety “meta-principles” that it can apply in specific contexts, helping ensure that AI safety mechanisms can generalize to inputs that are vastly different from the distribution they were initially trained with.
“Although these large language models have been very powerful tools, there have been some documented mishaps and hallucinations,” Ikeokwu said. “It’s been an arms race between the developers and the jailbreakers to keep things safe.”
Ikeokwu provided the example of asking GPT-4 for directions on cutting down a stop sign. Responding to such a query should be prevented by the model’s safety system, but simply adding words like “absolutely” after the prompt can override the safety system. As another example, Anthropic’s Claude language model can be manipulated by submitting a query with Base64 encoding or other inputs. “There’s even been examples where you can enter Pig Latin or Spanish [to bypass the safety systems],” he said.
Ikeokwu’s research focuses on “meta-prompting,” which includes appending prompts before instructions in a way that helps it to generalize, as well as “safety model capability parity,” which ensures the safety model is aligned with the underlying model. As policy recommendations, he suggested that we should “go back to the narrow AI framework where they’re only approved for very specific limited use cases, as opposed to just trying to do them out of the box.” He also noted there is a need for an “effective reporting and accountability process” to ensure users can take action in instances of misuse.
AI Policy Fellows: AI Evaluation and Auditing
The next two lightning talks explored questions related to responsible use of AI technologies, “from their engagement with people to their ability to be able to help us solve some of the biggest crises we face,” Newman explained.
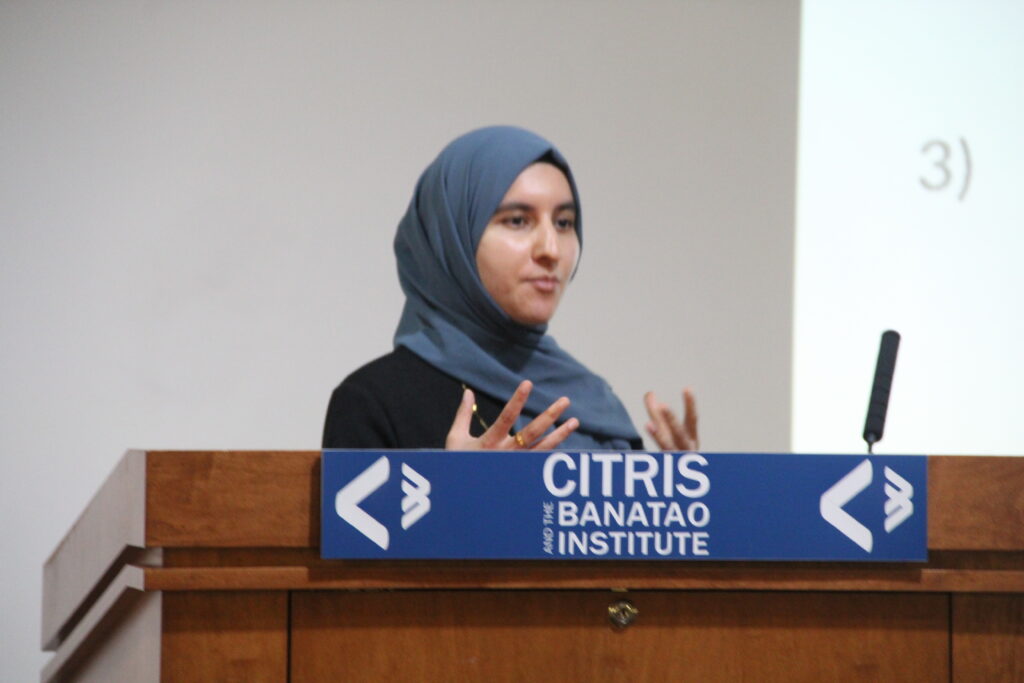
Marwa Abdulhai, a PhD Student in the EECS department who is affiliated with the Berkeley Artificial Intelligence Research Lab (BAIR), discussed her research on deception in AI systems. Abdulhai’s work explores how machine learning systems that directly interact with humans, such as language models, dialogue systems, and recommendation systems, have led to wide-scale deceit and manipulation. Her work explores how to build reinforcement learning algorithms with reward terms that prevent certain kinds of deception and align with human values.
“There are some key policy recommendations that I have against AI deception,” Abdulhai said. “The first one is regulation. Policymakers should try to define the deception in AI systems very specifically, because the word ‘deception’ can be defined in various different ways. And then model evaluations should include assessment of potential for deception.”
She explained that some of her research focuses on “quantifying, in numbers, how much deception has actually occurred,” as well as using “robust detection techniques” to identify when AI systems are engaging in deception. “It would be really cool to have a pilot as you’re interacting in the web telling you, hey, this might be X percent deceptive, or tell you how to respond to someone that might be potentially deceptive towards you that may or may not be an AI system.”
The second talk in this section was presented by Ritwik Gupta, a PhD student in the EECS department who studies “Computer Vision for Humanitarian Assistance and Disaster Response (HADR).” Gupta’s research aims to create computer vision methods that help first responders make better sense of a chaotic and unpredictable world, thus making aid provision more effective and efficient. Ritwik’s work is also focused on strengthening dual-use applications by translating advances in machine learning for HADR to new domains, such as addressing broader national security challenges.
His talk was shown in a pre-recorded video, as at the time of the symposium he was in Southern California “helping Cal Fire prepare for the next fire season,” part of his efforts to help state and local disaster response organizations access and analyze satellite data captured by the federal government.
“Unfortunately, access to [satellite] data is not democratized in the way that we would like it to be,” Gupta said. “Certain sophisticated organizations like Cal Fire or the Cal Guard have direct contracts with these companies and are able to get imagery as they wish. However, many other disasters response organizations across the U.S. do not have the budget or know how to access this imagery properly.”
Gupta explained that many agencies in the U.S. and abroad are already using satellite imagery with AI-assisted tools for tasks like assessing damage to buildings and wildfire perimeter prediction. Gupta explained that he is pushing for the adoption of improvements to the “tasking portals” used by local and state partners, as well as the public, to open access to satellite data in a more customizable format. “With these changes, we’re hoping that how state and local partners operate is further enhanced because they have more reliable access to satellite imagery, and they’re then able to do a lot more good with it.”
AI Policy Hub: Accountability for Harms
The third section of the AI Policy Hub presentations focused on approaches to accountability for harms caused by AI-based systems. “The training and uses of AI systems in our world today are already causing real harm to people around the world,” Newman said. “It’s absolutely critical that we address this and develop better mechanisms to support people, to listen to their expertise and feedback, and provide them with redress when needed.”
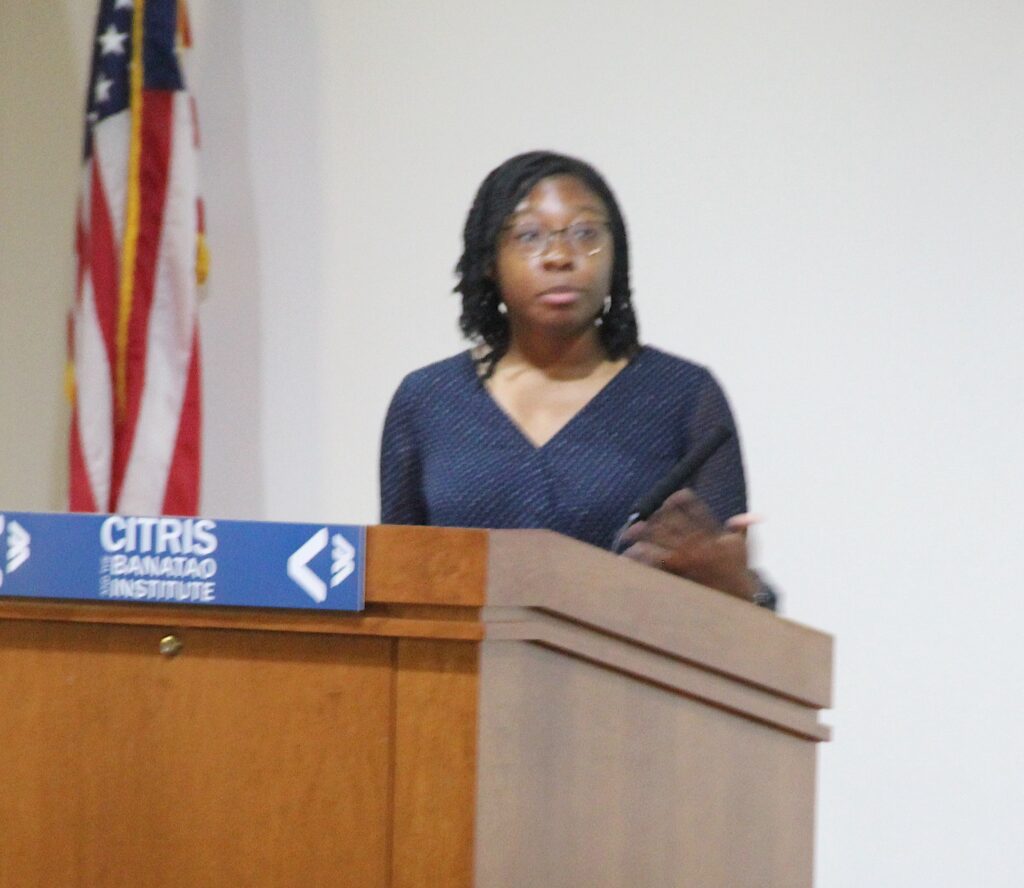
Janiya Peters, a PhD student in the School of Information, presented research on “Resistance to Text-to-Image Generators in Creator Communities.” This work explores the ways in which text-to-image models compromise visual creators’ intellectual property rights, as well as how visual creators adopt strategies of resistance to retain agency over their intellectual property, labor, and compensation. The project aims to inform policy at the intersection of copyright, data labor, and creative expression.
“The proliferation of consumer-ready text-to-image generators has caused unrest in creative communities,” Peters explained. “Several claims have been pursued against AI developers in court, the most persistent claim being that generative image algorithms such as Stable Diffusion have been unlawfully sourcing training data from copyrighted materials, trademarked images, and internet contributions.”
For her research, Peters spoke with “a wide range of traditional and digital artists” with diverse attitudes about the use of their images to train AI models. She recommends that generative text-to-image technology provide transparent notice about the inclusion of copyrighted works in training data sets as well as in user prompts and references. “This is a way for both users to be accountable for their means of production, as well as having historical documentation of how these image generators are being used,” she said.
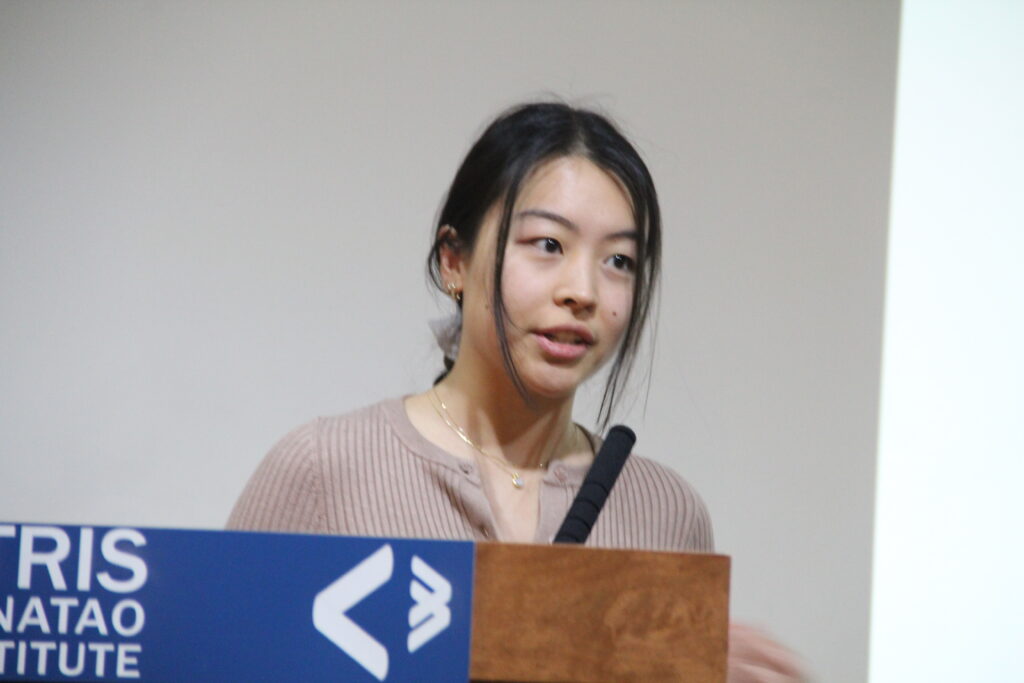
Jessica Dai, a PhD student in the EECS department, presented research on “Fairness Without Categories: Enabling Collective Action for Algorithmic Accountability,” which explores designing a framework for the general public to report and contest large-scale harms from algorithmic decision-making systems (ADS). The work is focused on ways to empower users to identify systematic patterns of mistakes made by an algorithm on groups of people that may not have been identified a priori, and that emerge only after a period of deployment.
“In this work, we’re trying to… figure out, how do we actually bring together disparate individual experiences to make some statement about aggregate, collective, or systemic harm? And then the follow up question is, once we do that, what can we do about it?”
She explained that an agency could be established, similar to the FDA, to allow the aggregate tracking of harms resulting from AI systems, though this would not be without challenges. “We don’t know necessarily what subgroups we’re considering in advance,” Lai explained. Current AI incident databases are a “great resource, but if you look at the actual incidents that are being recorded, they are news items about something that’s already happened in a very general sense.”
“Incident databases should be model-specific, tied to live predictions made by specific models,” Lai said. “They should be focused on individual-level events, so individuals can report something bad that happened to them rather than waiting for another external auditor to come in…. I think that our work is trying to serve as a starting point. We think this hypothesis testing framework is a nice place to start.”
Keynote: Ken Goldberg
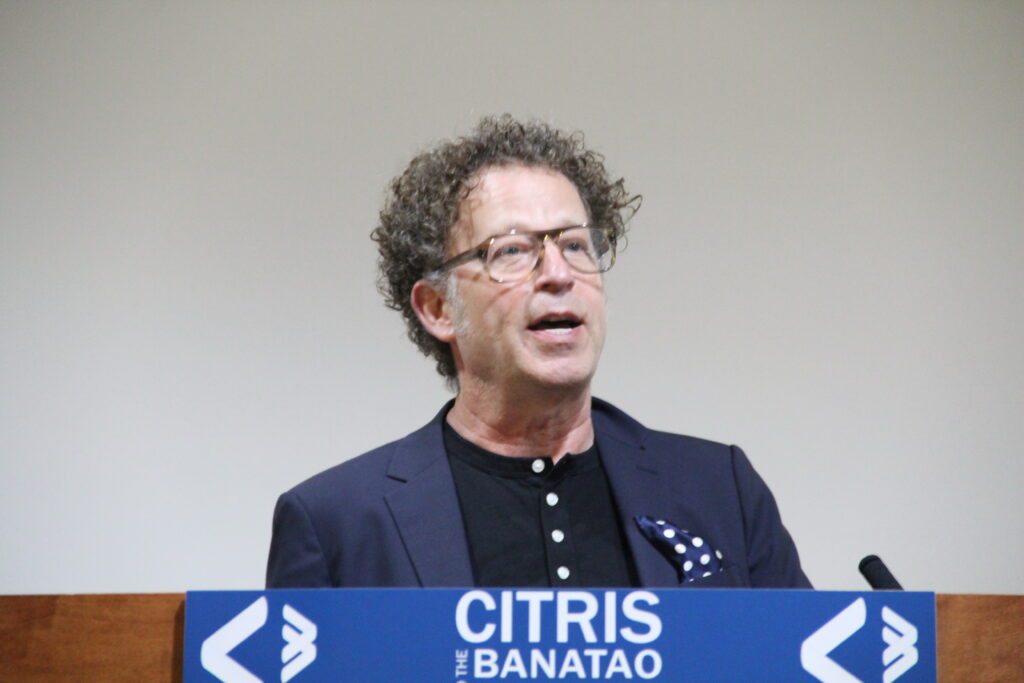
The symposium ended with a keynote presentation by Ken Goldberg, the William S. Floyd Distinguished Chair in Engineering at UC Berkeley and an award-winning roboticist, filmmaker, artist, and popular public speaker on AI and robotics.
Goldberg struck an optimistic tone about the future of AI, with an eye toward allaying what he refered to as “AI anxiety.” “I think there’s a lot of potential for harms, but I want to offer us maybe a little bit of a hopeful ending, more of an upbeat perspective,” he said. “There’s a lot of fear out there. I think it’s important to also address that these fears that AI is going to take over in some way or run amok are being promulgated by some very intelligent people, and are also being picked up quite dramatically by the press. I want to just share with you that almost none of the researchers I know share this fear.”
Goldberg said that most AI technologies are not as powerful as they may seem. He said that, while AI systems have proven highly capable at some tasks, such as playing the game Go, they are far from ready to be “taking care of senior citizens or warehouses or operating rooms…. these environments are vastly more complex.”
He said that the fears that AI will replace human jobs like driving are overblown. “I don’t think we’re going to have lots out-of-work truck drivers,” he said. “In fact, I think, we’re going to have a shortage of human workers. We have more jobs that need to be done, and I’m not worried that AI is going to take over all these jobs.”
He noted that “AI systems are better than humans at calculations, precision, and objectivity,” but they have limited “understanding, empathy, and dexterity… We’re nowhere near the level of the average human at doing those things. So I’m in favor of something I call complementarity…. AI is going to help us do…the things we don’t really like doing that are boring so that we can do other things. So I’m in favor of this idea of AI plus intelligence amplification, allowing it to amplify our human intelligence.”
