Consider an organization that collects sensitive information about individuals, and needs to share the results of analyzing that data while respecting the individuals’ privacy. The US Census Bureau, for example, collects detailed records on hundreds of millions of individuals, and is mandated to share as much useful aggregate information as possible subject to individual confidentiality. This general problem is now ubiquitous—it is faced by government agencies (the IRS and CDC, for instance), consumer technology providers (such as Google, Apple, and Facebook), healthcare providers, and educational institutions, among others. Statistical disclosure limitation is an old field, but the past two decades have seen numerous demonstrated failures of traditional statistical disclosure limitation paradigms, most notably “de-identification” and naive anonymization. The increasing rate and scope of personal data collection makes it challenging to reason about the information leaked by a particular release. Driven by this challenge, a rigorous foundational approach to private data analysis has emerged in theoretical computer science in the last decade, with differential privacy and its close variants playing a central role. The resulting body of theoretical work draws on many scientific fields: statistics, machine learning, cryptography, algorithms, databases, information theory, economics and game theory. This research has now influenced how privacy scholars think and argue about privacy, and how sensitive data are treated in real-life applications. This ongoing process reinforces the need for further technical research on foundations of privacy and understanding of the technical, legal, social and ethical issues that arise. This program aims to advance core research on privacy and to foster new collaborations between researchers who work on theoretical aspects of data privacy and those working in areas of potential applications.
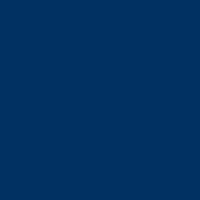
Grant /
January 2020