In the past year, several major companies have deployed data processing systems based on differential privacy. These systems use mathematical techniques to limit the information that can be learned about individuals, but a number of limitations threaten the success of this approach. This research will bring statistical techniques to bear on private algorithms, expanding the range of scenarios for which meaningful privacy guarantees can be supported, and providing a theoretical basis to understand related privacy standards. These techniques will be applied in a prototype deployment of a provably private database.
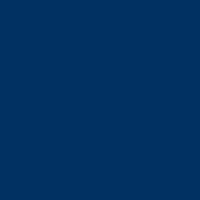
Grant /
January 2020