Providing improved attack probability estimation is a tangible and useful way to shore up cyberdefenses for individual, technological, and societal systems. This research is aimed at developing a data-driven estimation framework for assessing the probabilities of diverse cybersecurity threats. The researchers are developing algorithms for estimating threat probabilities that are model agnostic, i.e. without a presumption that the kinds of attacks are known, meaning they can estimate new and as yet unseen attacks.
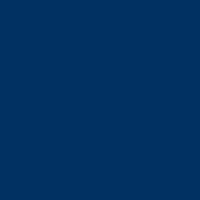
Grant /
January 2020